- February 29th, 2024
- Category: news
From Images to Impact: Radiomics' Solutions to Challenges in Immunotherapy
Up to 30% of patients may experience hyper progression following immunotherapy. Identifying patients who will actually benefit from immunotherapy is intrinsic to achieving optimal outcomes. Radiomics can offer a solution to this problem by analysing the phenotypical changes in lesions and organs that may occur following the initiation of a treatment.
Blogpost
From Images to Impact: Radiomics' Solutions to Challenges in Immunotherapy
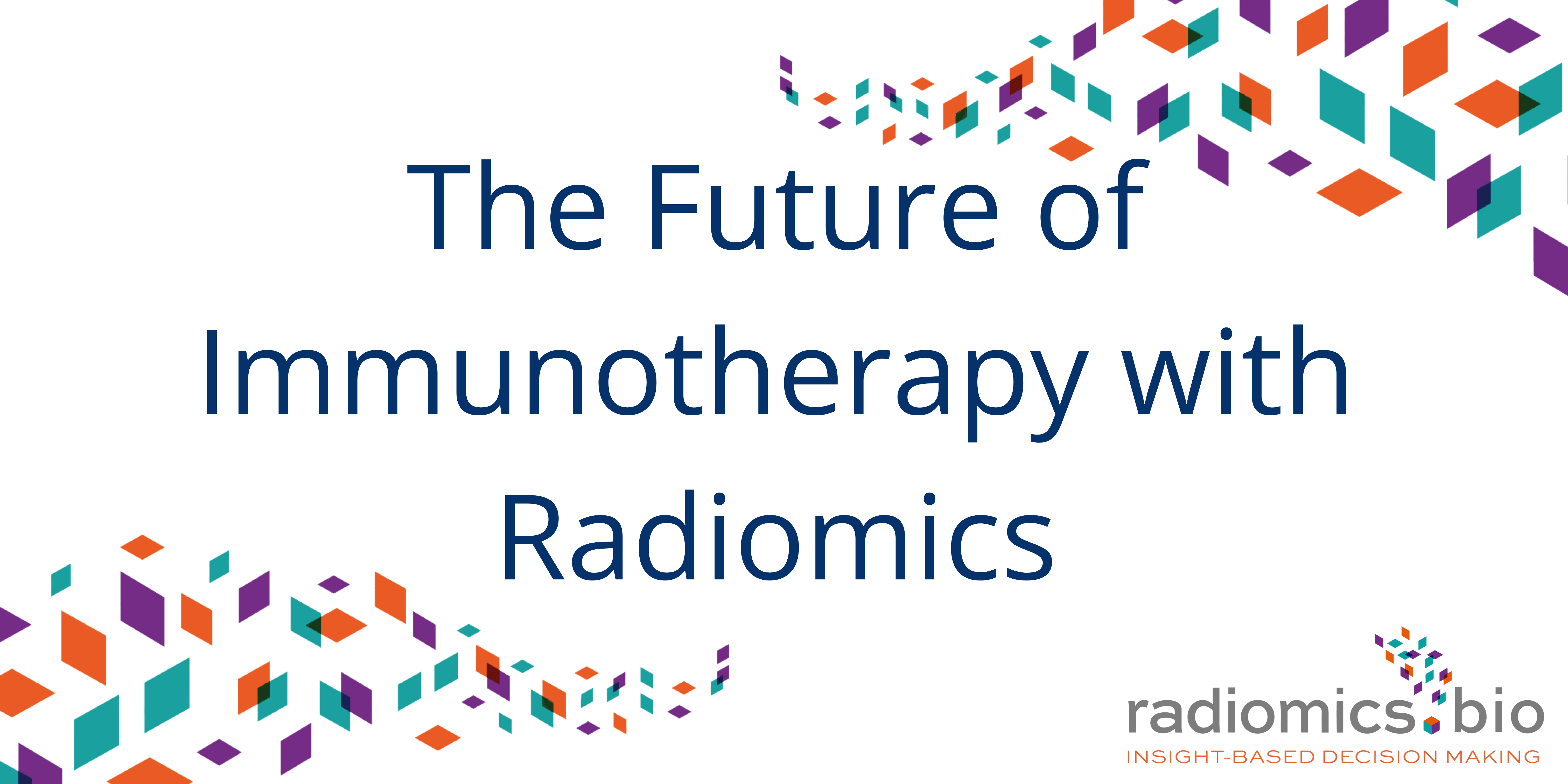
Cancer immunotherapy, harnessing the body's immune system to fight malignant cells, represents a groundbreaking shift in the oncology landscape and has marked a turning point in cancer treatment achieving remarkable results in patients. In recent years, immunotherapy has emerged as the therapeutic option of choice for several advanced cancers. However, accurate assessment of treatment response in immunotherapy is crucial. Unlike traditional chemotherapy, immunotherapy may not exhibit immediate tumour shrinkage, rendering conventional response criteria inadequate. The integration of Radiomics and Artificial Intelligence (AI) has the potential to revolutionise the field of immunotherapy response assessment, offering a promising avenue to enhance cancer treatment strategies.
Radiomics is a quantitative imaging process extracting and analysing high quantities of digital information from standard-of-care imaging. This new wealth of data opens the door to increased analytics and the possibility of building artificial intelligence-based models to assess clinical outcomes, including prognostic or prediction of response to treatment (1). Radiomic models can also be used to characterise the genotype of tumours, overall survival, the response of a disease to a given treatment or to assess the response to a treatment beyond the classic radiologic criteria (2).
As the effectiveness of immunotherapy varies among patients and especially among lesions, making precise local response assessments is crucial. With its ability to analyse vast datasets swiftly and accurately, AI could play a pivotal role in this endeavour. This blog post delves into the possible role of radiomics and advanced imaging analysis in evaluating immunotherapy responses, highlighting their importance in guiding clinical decisions and optimising patient outcomes.
Imaging plays a fundamental role in modern cancer management. It provides a non-invasive method to visualise tumours, assess their size, and monitor changes over time. Traditional modalities such as CT, MRI, and PET have been invaluable in tracking cancer progression (3). Furthermore, the emergence of specialised imaging techniques like Immuno-PET have opened new frontiers in assessing immunotherapy responses (4). These technologies offer insights into tumour biology, immune infiltration, and treatment-induced changes, facilitating a more comprehensive evaluation of therapeutic efficacy. Immunotherapy introduces unique complexities in response assessment. Pseudo progression, where tumours initially appear to grow before responding to treatment, can complicate interpretation (5). Conversely, in hyper progression an unexpected rapid progression in disease following immunotherapy can be registered (6). However, a significant challenge is posed by the heterogeneity within and between tumours, as some lesions may respond while others progress (7). Determining the optimal timing and frequency of assessments remains an ongoing issue. Addressing these challenges is vital for accurate response evaluation, ensuring that patients receive timely adjustments to their treatment plans.
Many drugs have received regulatory clearance both in the EU and US for melanoma, lung, bladder, renal and oropharyngeal cancers among others (8). In turn, the tools to assess therapy efficacy and response have evolved to better understand the disease progression and the effects of these new therapies. This is the case for example with RECIST criteria, originally developed for chemotherapy, was updated with immune-related response criteria (irRC), immune-related RECIST (irRECIST), and immune RECIST (iRECIST) (3). Image assessment of cancerous lesions development is still the state of the art for therapy planning and follow-up, but novel tools are required to improve patient management and quality of life.
The integration of radiomics and AI has the potential to revolutionise the field of immunotherapy response assessment, offering a promising avenue to enhance cancer treatment strategies. Immunotherapy effectiveness varies among patients and among lesions, making precise response assessment crucial. With its ability to analyse vast datasets swiftly and accurately, AI could play a pivotal role in this endeavour. AI algorithms can process a multitude of quantitative features extracted from radiological images, collectively known as radiomics features (9). These features encompass information about tumour heterogeneity, shape, and texture, which may hold the key to predicting immunotherapy response as well tumour biology.
Through machine learning models, AI can correlate radiomics features with clinical outcomes, helping clinicians stratify patients into different response categories or to predict the insurgence of immune-related adverse events. Moreover, radiomics can expedite the assessment process, enabling timely adjustments to treatment plans and reducing the burden of unnecessary side effects for non-responsive patients. This in turn could also improve the cost-effectiveness of the drug development life cycle, reducing the rate of failed clinical trials in oncology and costs for pharmaceutical companies.
Radiomics in practice:
Correlation of radiomic features with tumour biology
The field of radiomics offers additional information for the prediction of prognosis in patients treated with immunotherapy based on established molecular biomarkers. For example, Chen et al. (10) assessed the ability of radiomic features to predict tumour-infiltrating lymphocytes (TIL) status of a tumour in patients with hepatocellular carcinoma (HCC). Features extracted from MRI tumoral and peri-tumoral region were used to predict the immunoscore of patients, which assessed the density of CD3+ and CD8+ T cells within the tumour core and invasive margin. This study found that a radiomics model which incorporated both intra and peri-tumoral radiomic features had a higher AUC in the validation cohort compared to a model with tumoral features alone (0.89 vs. 0.63).
Several studies have already explored the association of radiomic features with PD-L1 status (11),(12),(13). One of the first studies assessing the ability of radiomic features to predict PD-L1 status was conducted by Jiang et al.(14) This was a retrospective single-centre study that extracted radiomic features from both the CT and PET scans of a pre-operative PET/CT in patients with lung cancer undergoing surgery. The PD-L1 status of patients was assessed on postoperative samples via immunohistochemistry testing. The model performed very well for both PD-L1 ≥ 1% and PD-L1 ≥ 50%. A significant strength of this study is the use of post-operative samples to detect PD-L1 status. Another study considering patients with Stage IV NSCLC, found a radiomics-based model predictive of PD-L1 ≥ 50% on biopsy samples with moderate performance (AUC 0.65) (15).
Other tissue biomarkers of interest include microsatellite instability (MSI) and tumour mutational burden (TMB) (13), (16). One study was able to validate a radiomics model incorporating 40 radiomic features to predict microsatellite instability in colon cancer with an AUC of 0.76 (17). Another study in patients with NSCLC developed both a CT-based radiomics and a deep learning model to predict TMB (AUC 0.74 and AUC 0.85, respectively) (18).
Prediction of immune-related adverse events
Several studies investigated the ability of radiomics to predict immune-related adverse events. Mu et al. were able to train and validate a radiomics model derived from PET/CT features of the primary tumour in patients with NSCLC undergoing ICI treatment to predict the risk of immune-related severe adverse events (19). The model also incorporated clinical features including type and dose of immunotherapy. Another study, analysing a mixed cohort of patients, developed a radiomics model based on CT-derived features from the lung to predict the risk of pneumonitis with 100% accuracy, however the number of patients in the study was quite small, with only 2 out of 32 patients experiencing pneumonitis (20). Some research has also been carried out on radiomics-based distinction between radiation and immune-induced pneumonitis (21), (22). This is a very promising avenue of research, tackling a highly relevant unmet clinical need.
Predicting response to immunotherapy
Individual patient response to specific therapy is a fundamental aspect of radiomics potential. Radiomics.bio conducted a retrospective study to determine the role of CT-based radiomics and delta-radiomics in predicting tumoral response and survival in patients with advanced NSCLC treated with PD-1/PD-L1 inhibitors (1).
Delta radiomics extracts radiomic features from the same region of interest of the same patient at different time intervals to study the variation of radiomic features over time. Studying the phenotypical modifications of tissues and or lesions that may occur following the introduction of treatment before any size modification bears a significant promise in correctly assessing immunotherapy, wherein the kinetics of tumour size is not always correlated to treatment response.
Our results, illustrated in Figure 1.0 below, demonstrated the ability of a CT-based delta-radiomics signature to perform early identification of patients who are more likely to benefit from PD-1/PD-L1 inhibitors. Thus, further confirming the ability of radiomics to provide a solution for the challenges encountered in the development of immunotherapies. Radiomics.bio’s delta-radiomics signature was able to identify patients who presented a clinical benefit at 6 months, with an AUC obtained on an external test dataset of 0.8 (95% CI: 0.65-0.95)(1).
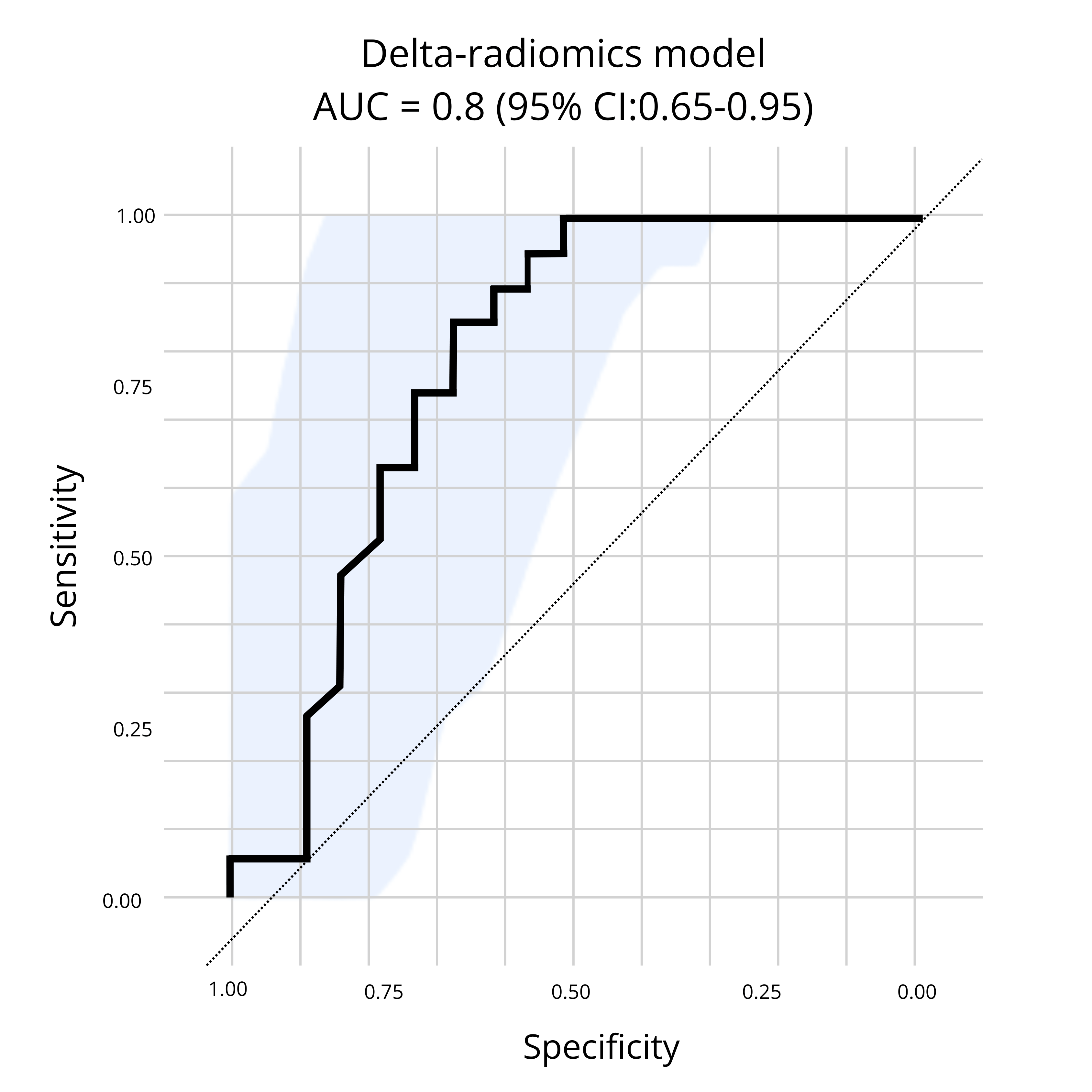
Figure 1: Presented at SITC,Radiomics.bio’s delta-radiomics signature demonstrates the ability to identify patients who presented a clinical benefit at 6 months, with an AUC obtained on an external test dataset of 0.8 (95% CI: 0.65-0.95), from baseline + first follow up CT scan.
Conclusion
Radiomics complements other modes of analysis by filling in gaps of knowledge through additional insights, moreover, the radiomic solution proposes multiple advantages including being non-invasive, repeatable over time and being able to explore the entirety of the primary tumour as well as all secondary lesions included in medical scans (1). By identifying quantitative features that are not identifiable by simple visual inspection, radiomics creates enhanced data models to allow for insight-based decision making (2).
About Radiomics
Radiomics is an imaging research organisation which focuses on AI powered healthcare, with a unique expertise in Radiomics, Deep Learning & Federated Learning applied to oncology and other therapeutic areas. Founded in 2016, Radiomics leverages its proprietary technology to support pharma and biotech companies in all phases of clinical development to extract the maximum information from clinical trial images. Based in Liège, Belgium, Radiomics offers advanced image analysis services to support both prospective and retrospective clinical trials to better understand observed treatment effect.
With collaborations in place with several hospitals and pharma companies, Radiomics is dedicated to its mission to enable the right diagnosis & treatment for the right patient through quantitative imaging and machine learning.
References
1. Cousin F, Louis T, Dheur S, Aboubakar F, Ghaye B, Occhipinti M, et al. Radiomics and Delta-Radiomics Signatures to Predict Response and Survival in Patients with Non-Small-Cell Lung Cancer Treated with Immune Checkpoint Inhibitors. Cancers. 2023 Jan;15(7):1968.
2. Porcu M, Solinas C, Mannelli L, Micheletti G, Lambertini M, Willard-Gallo K, et al. Radiomics and “radi-…omics” in cancer immunotherapy: a guide for clinicians. Crit Rev Oncol Hematol. 2020 Oct 1;154:103068.
3. Berz AM, Dromain C, Vietti-Violi N, Boughdad S, Duran R. Tumor response assessment on imaging following immunotherapy. Front Oncol. 2022;12:982983.
4. Dercle L, Sun S, Seban RD, Mekki A, Sun R, Tselikas L, et al. Emerging and Evolving Concepts in Cancer Immunotherapy Imaging. Radiology. 2023 Jan;306(1):32–46.
5. Frelaut M, du Rusquec P, de Moura A, Le Tourneau C, Borcoman E. Pseudoprogression and Hyperprogression as New Forms of Response to Immunotherapy. BioDrugs. 2020 Aug 1;34(4):463–76.
6. Sehgal K. Hyperprogression in Patients With Cancer Receiving Immune Checkpoint Inhibitors. JAMA Netw Open. 2021 Mar 1;4(3):e211839.
7. Pseudoprogression and hyperprogression after checkpoint blockade - ScienceDirect [Internet]. [cited 2024 Feb 22]. Available from: https://www.sciencedirect.com/science/article/abs/pii/S1567576918301218
8. Diseases | Free Full-Text | Emerging Trends in Immunotherapy for Cancer [Internet]. [cited 2024 Feb 22]. Available from: https://www.mdpi.com/2079-9721/10/3/60
9. Guiot J, Vaidyanathan A, Deprez L, Zerka F, Danthine D, Frix AN, et al. A review in radiomics: Making personalized medicine a reality via routine imaging. Med Res Rev. 2022;42(1):426–40.
10. Chen S, Feng S, Wei J, Liu F, Li B, Li X, et al. Pretreatment prediction of immunoscore in hepatocellular cancer: a radiomics-based clinical model based on Gd-EOB-DTPA-enhanced MRI imaging. Eur Radiol. 2019 Aug 1;29(8):4177–87.
11. Frontiers | Radiomics Study for Predicting the Expression of PD-L1 and Tumor Mutation Burden in Non-Small Cell Lung Cancer Based on CT Images and Clinicopathological Features [Internet]. [cited 2024 Feb 22]. Available from: https://www.frontiersin.org/journals/oncology/articles/10.3389/fonc.2021.620246/full
12. Biomedicines | Free Full-Text | Multi-Omics Approaches for the Prediction of Clinical Endpoints after Immunotherapy in Non-Small Cell Lung Cancer: A Comprehensive Review [Internet]. [cited 2024 Feb 22]. Available from: https://www.mdpi.com/2227-9059/10/6/1237
13. Cancers | Free Full-Text | NSCLC Biomarkers to Predict Response to Immunotherapy with Checkpoint Inhibitors (ICI): From the Cells to In Vivo Images [Internet]. [cited 2024 Feb 22]. Available from: https://www.mdpi.com/2072-6694/13/18/4543
14. Assessing PD-L1 Expression Level by Radiomic Features From PET/CT in Nonsmall Cell Lung Cancer Patients: An Initial Result - ScienceDirect [Internet]. [cited 2024 Feb 22]. Available from: https://www.sciencedirect.com/science/article/abs/pii/S1076633219302338
15. Tian P, He B, Mu W, Liu K, Liu L, Zeng H, et al. Assessing PD-L1 expression in non-small cell lung cancer and predicting responses to immune checkpoint inhibitors using deep learning on computed tomography images. Theranostics. 2021 Jan 1;11(5):2098–107.
16. Lin ZF, Qin LX, Chen JH. Biomarkers for response to immunotherapy in hepatobiliary malignancies. Hepatobiliary Pancreat Dis Int. 2022 Oct 1;21(5):413–9.
17. Golia Pernicka JS, Gagniere J, Chakraborty J, Yamashita R, Nardo L, Creasy JM, et al. Radiomics-based prediction of microsatellite instability in colorectal cancer at initial computed tomography evaluation. Abdom Radiol N Y. 2019 Nov;44(11):3755–63.
18. He B, Dong D, She Y, Zhou C, Fang M, Zhu Y, et al. Predicting response to immunotherapy in advanced non-small-cell lung cancer using tumor mutational burden radiomic biomarker. J Immunother Cancer. 2020 Jul 6;8(2):e000550.
19. Mu W, Tunali I, Qi J, Schabath MB, Gillies RJ. Radiomics of 18F Fluorodeoxyglucose PET/CT Images Predicts Severe Immune-related Adverse Events in Patients with NSCLC. Radiol Artif Intell. 2020 Jan;2(1):e190063.
20. Colen RR, Fujii T, Bilen MA, Kotrotsou A, Abrol S, Hess KR, et al. Radiomics to predict immunotherapy-induced pneumonitis: proof of concept. Invest New Drugs. 2018 Aug 1;36(4):601–7.
21. Differentiation between immune checkpoint inhibitor‐related and radiation pneumonitis in lung cancer by CT radiomics and machine learning - Cheng - 2022 - Medical Physics - Wiley Online Library [Internet]. [cited 2024 Feb 22]. Available from: https://aapm.onlinelibrary.wiley.com/doi/full/10.1002/mp.15451
22. Chen X, Sheikh K, Nakajima E, Lin CT, Lee J, Hu C, et al. Radiation Versus Immune Checkpoint Inhibitor Associated Pneumonitis: Distinct Radiologic Morphologies. The Oncologist. 2021 Oct 1;26(10):e1822–32.